标题
RaFe: Ranking Feedback Improves Query Rewriting for RAG
总结
本网页主要介绍了一种名为RaFe的查询重写框架,用于提高基于大型语言模型(LLMs)的检索增强生成(RAG)系统在开放领域问答(ODQA)任务中的性能。
摘要
RaFe框架通过利用公开可用的重排器提供反馈,有效地训练查询重写模型,而不需要额外的标注数据。该框架包括两个阶段:初始监督微调(SFT)和反馈训练。在SFT阶段,模型通过标准的监督微调获得重写能力。在反馈训练阶段,利用重排器的排名得分作为自然的反馈信号,通过离线和在线的强化学习(RL)方法进行训练。实验结果表明,RaFe在多个跨语言数据集上的表现优于基elines,特别是在EXPAND-Ranked设置下,其在问答任务中显示出显著的改进。此外,研究还探讨了不同数量的查询重写对RAG系统性能的影响,并指出在实际应用中,2-3个重写可能是最佳的平衡点。
观点
- 当前查询重写方法存在局限性: 目前的查询重写方法通常依赖于标注数据或特定设计的奖励函数,这限制了它们的泛化能力,并且无法充分利用针对查询重写的有效信号。
- RaFe框架的优势: RaFe通过引入基于重排器反馈的训练方法,克服了现有方法的限制,提供了一种更为通用和高效的查询重写训练方式。
- 反馈机制的重要性: 通过实验验证,RaFe利用重排器提供的反馈信号,能够显著提高查询重写的质量,进而提升RAG系统在ODQA任务中的性能。
- 查询重写数量的影响: 研究指出,查询重写的数量会影响RAG系统的响应时间和性能。实验结果表明,大约2-3个重写能够在性能和效率之间取得最佳平衡。
- 未来工作方向: 作者提出,未来的工作可能会集中在联合训练重排器和重写模型,以及探索如何在特定领域中利用RaFe框架。
Why
In this paper we attempt to
(i) reduce the cost of annotations for feedback; and
(ii) identify a signal that better aligns with the objectives of the query rewriting task.
Contribution
The main contributions of our paper can be summarized as follows:
- We propose RaFe, a novel query rewriting framework that utilizes feedback from the reranker, an especially fitting signal for the objective of retrieving more relevant documents.
- RaFe does not necessitate annotated labels or particularly designed scores, ensuring the generalizability of the training framework.
- We validate the effectiveness of our proposed approach on cross-lingual datasets across wide settings with a general and public reranker, we further conduct a comprehensive investigation of what makes a better query rewriting and how ranking feedback works.
How
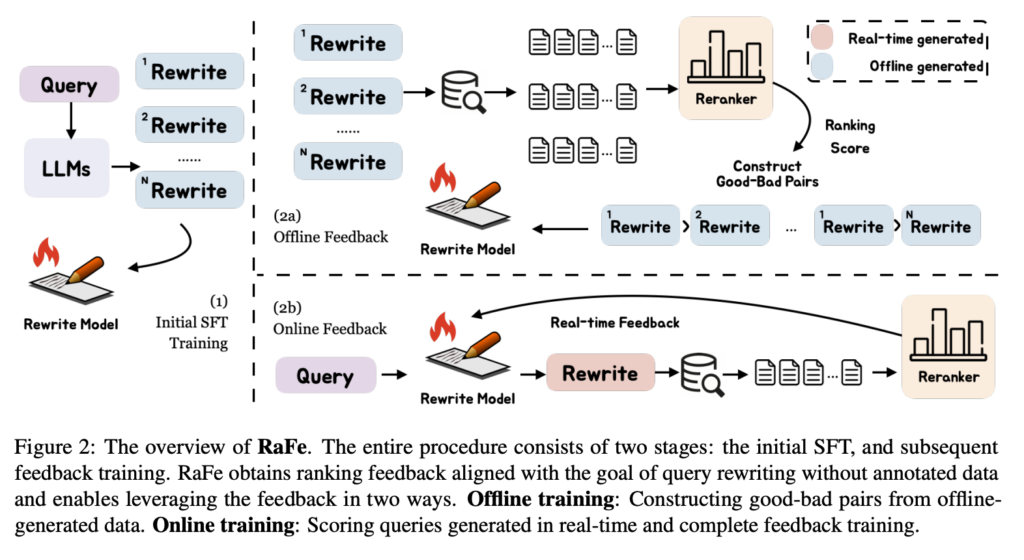
Result
RaFe rewrite 框架的效果略胜于 OQR、SFT 等其他方案。而标注成本极低
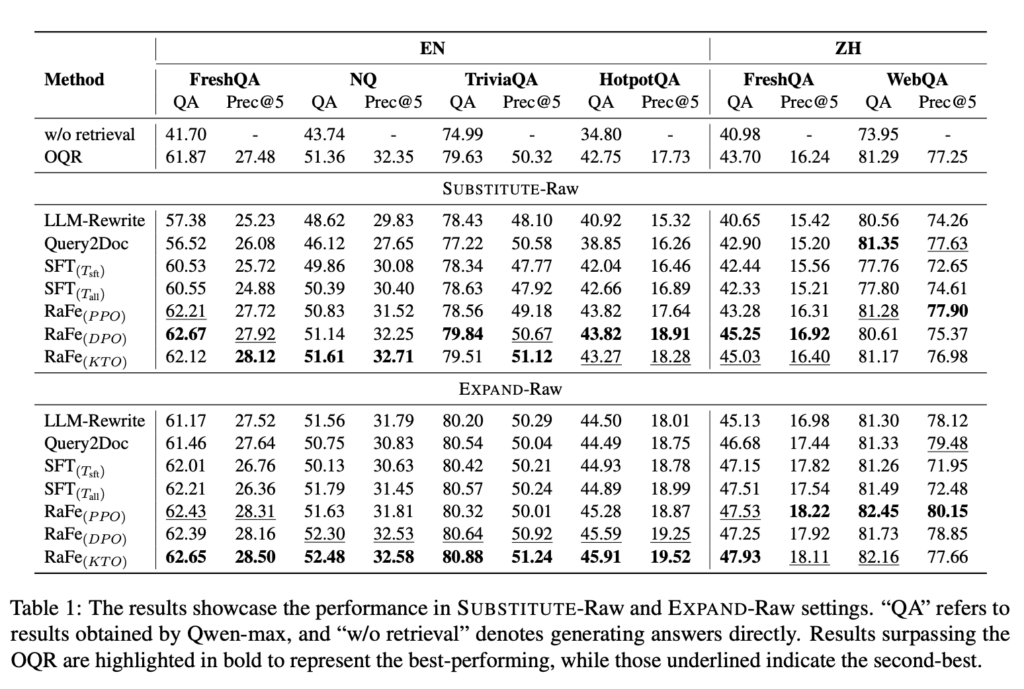
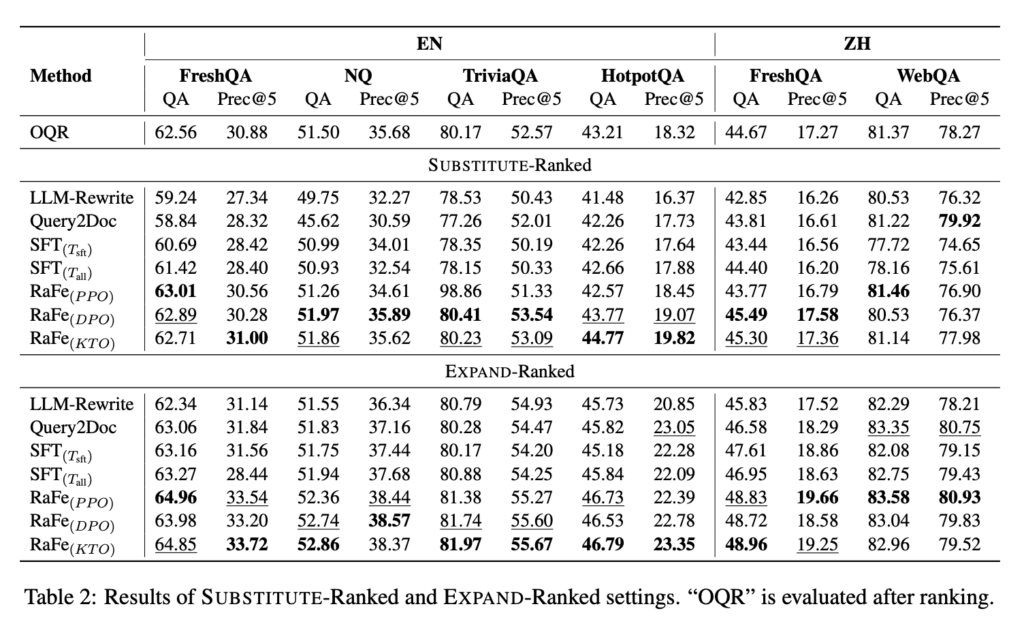
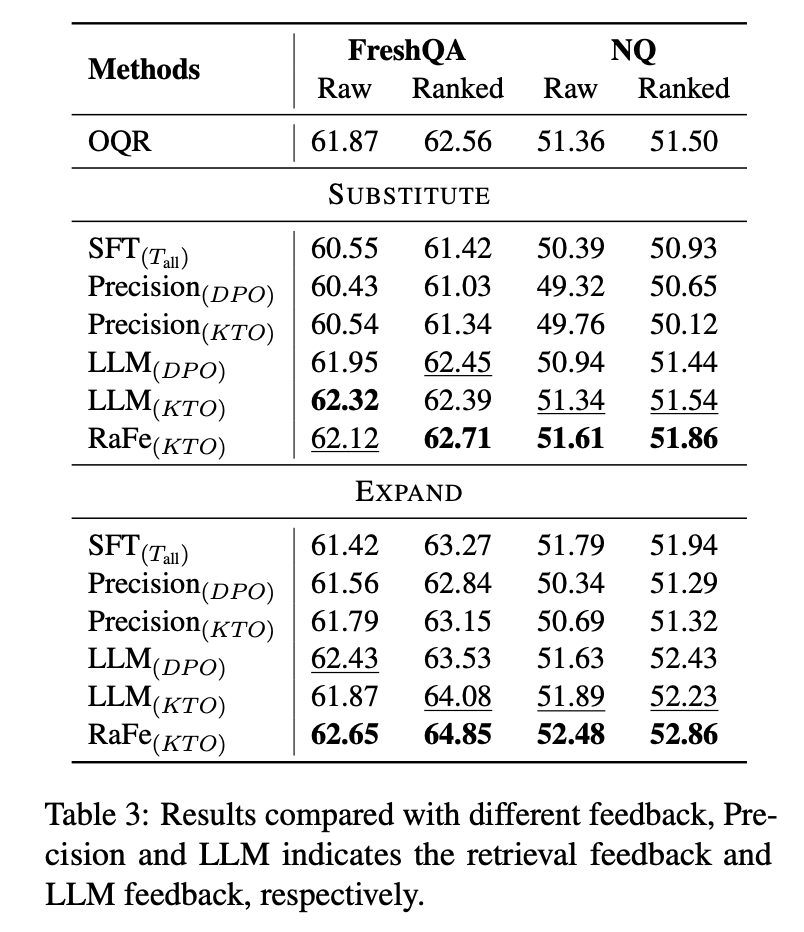
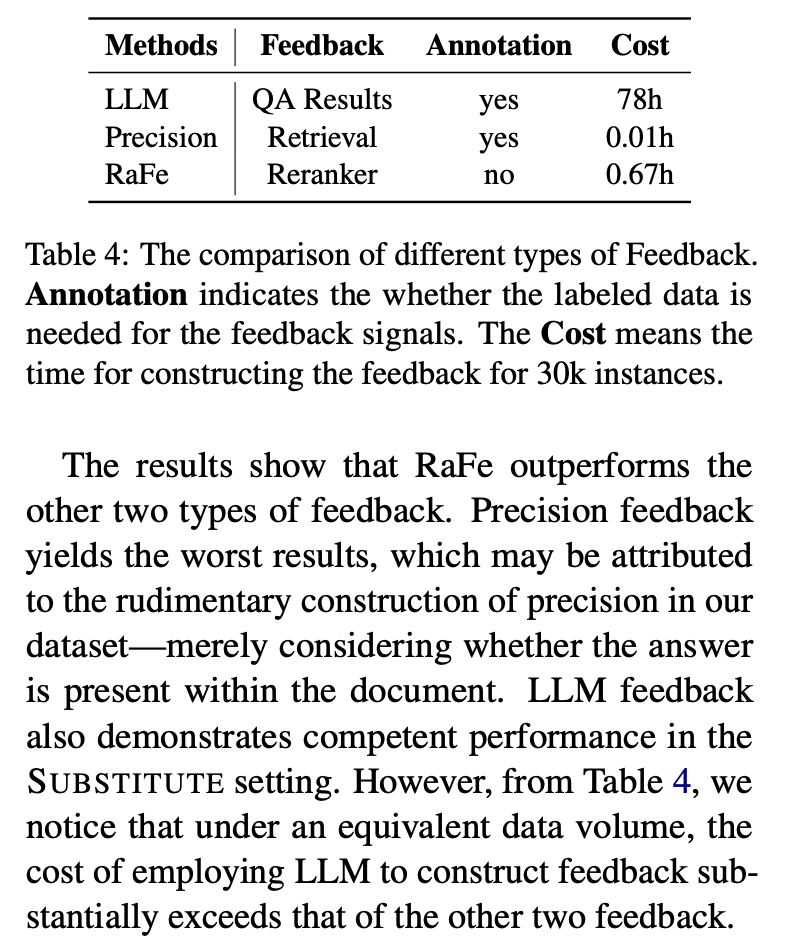
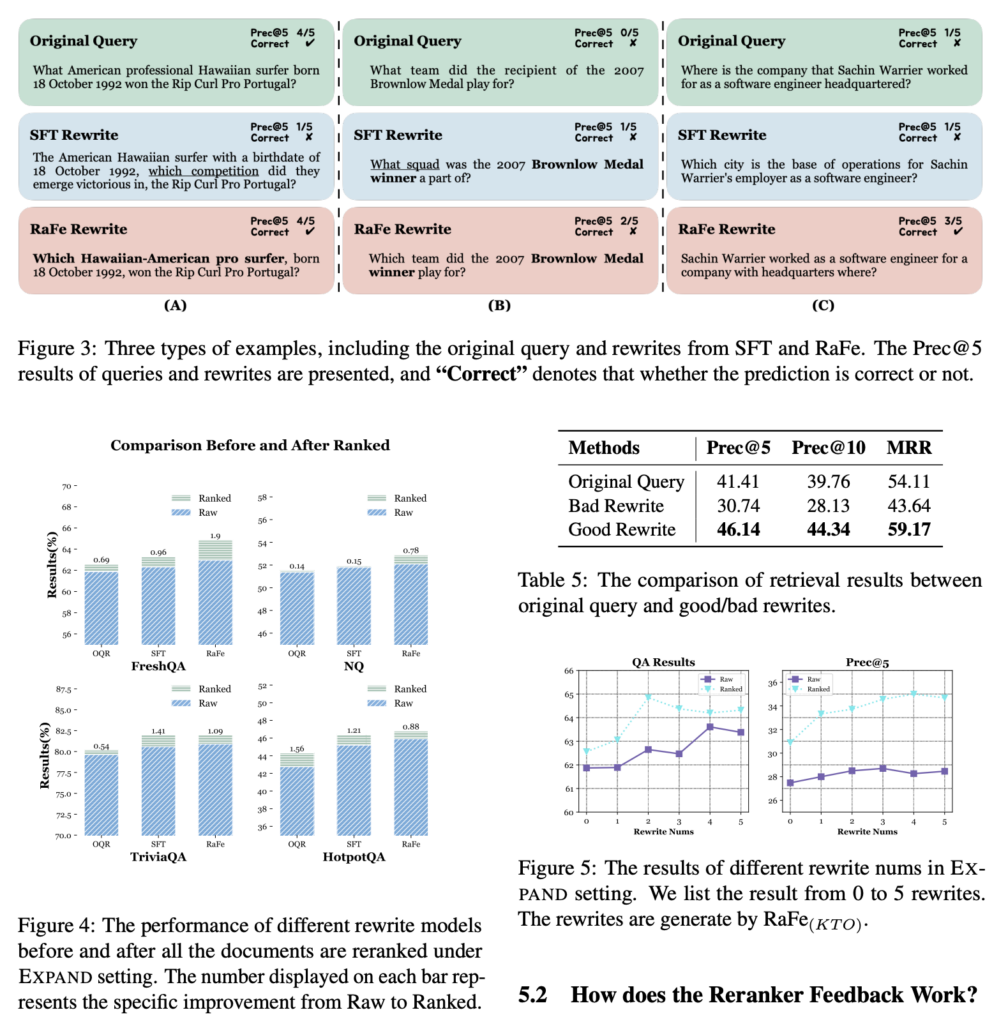
Leave a Reply